Big Data Analytics: Is Consent Required?
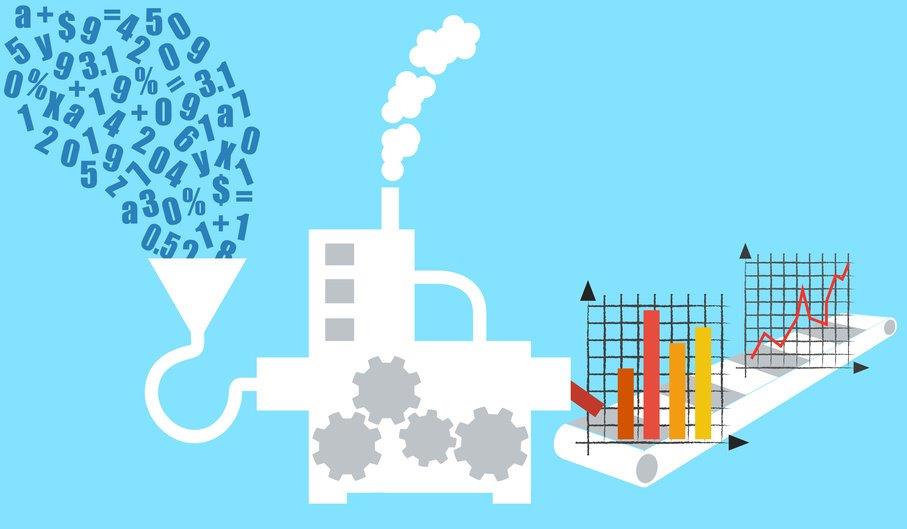
The panel discussed how analytics projects get authorized within organizations. We discussed two findings issued by the OPC in the last few years which pertain to data analytics in the context of advertising: PIPEDA Report of Findings #2015-001 (Bell’s Relevant Ads Program) and PIPEDA Case Summary #2009-004 (No Consent Required for Using Publicly Available Personal Information Matched with Geographically Specific Demographic Statistics).
We referred to recent data analytic reports that have been published in Canada as well as in the U.S. In Canada, we referred to the 2012 OPC report “The Age of Predictive Analytics: From Patterns to Predictions“. In the U.S., we discussed the January 2016 FTC Report “Big Data: A tool for Inclusion or Exclusion” as well as the more recent (May 2016) White House Report “Big Data: A Report on Algorithmic Systems, Opportunity, and Civil Rights“. We exchanged ideas on the main legal and privacy challenges when conducting business analytics, and when personal information is or should be considered as fully anonymized under applicable Canadian and U.S. laws.
We also discussed whether individuals should be entitled to consent to their information being used for new analytic purposes and if so, what type of consent should be obtained and in which situations. I had a few slides on the challenges when obtaining consent, in which I proposed different data flow scenarios. When we discuss big data analytics and privacy / consent issues, we could be discussing different situations which could raise very different risks and challenges. My proposed scenarios are a work in progress, in the sense that they are a first attempt at categorizing these different scenarios and identifying the risks. I had already presented these slides at an academic event which took place in Montreal last March.
The 3 scenarios that I propose are the following ones:
Scenario no. 1: Under this scenario, personal information is collected from an individual. It is then analyzed by the organization and the resulting (analyzed) data is then used towards the same individual.
Consent would be required under this scenario, although depending on the sensitivity of the information and the “reasonable expectation” of the individual, consent may be implied. Under PIPEDA Report of Findings #2015-001 (Bell’s Relevant Ads Program), the OPC articulated the view that express consent was required. I have discussed some of my concerns with this finding elsewhere.
Scenario no. 2: Under this scenario, personal information is collected from an individual. It is then analyzed in aggregate by the organization to obtain trends and knowledge. The trends (i.e. aggregated data) may be used internally or made available to third parties (but they are not used towards the individual).
Consent would only be required if we take the position that the process of analyzing the data (while aggregating the data) is a “new use” of information for which consent is required.
I would argue that consent should not be required under this scenario (or at least that consent may be implied) if: (i) the data minimization principle is complied with (the organization is not collecting more information than required for the primary service); and (ii) an acceptable level of aggregation is used (in order to be able to make the case that the information is no longer “personal information”) and this, even if PIPEDA does not specifically exclude the aggregation activity from the scope of the law.
There are different distinctions that could be made under this scenario: whether the organization is using the trends for internal purposes versus selling them to third parties, the use that will be made of these trends (there may be ethical concerns, etc.), whether the analysis conducted is in connection with the primary and core business activities of the organization (although many organizations’ business model will evolve over time, for instance telecommunication companies that expand their service offering to offer health services, etc.). At the end of the day, my concern is that requiring consent (and more specifically express consent) to allow organizations to conduct this type of activity would hamper innovation. I think that, perhaps, the privacy risks may be caught through scenario 3, at the point where the trend is then used towards an individual.
Scenario no. 3: Under this scenario, trends are obtained from third parties (for instance, studies’ results, statistics, scores or trends may be obtained from analytic companies). The trend is then linked to a profile and applied towards an individual (used in a decision pertaining to a specific individual).
Some may argue that since a “trend” (or a statistic, etc.) is not necessarily personal information, no consent should be required under this scenario. In PIPEDA Case Summary #2009-004, public directory information was merged with non-personal aggregated geodemographic information. The OPC found that no consent was required as it did not regard the fact that a person lives in a neighbourhood with certain characteristics to be personal information about the individual (it was considered as information about the neighbourhood, not about the individual).
I would argue that whether consent should be required under this scenario 3 depends on the use that will be made of the data. While I am much less concerned with data being used for advertising purposes (such as under the PIPEDA Case Summary #2009-004 discussed above), perhaps the risks are greater when data is used in eligibility decisions (employment, housing, access to financial products, admission to schools, etc.). For example, a credit card company lowering a customer’s credit not based on his/her payment history, but based on other customers with a poor repayment history, simply because they have shopped at the same establishment or because they live in a similar neighbourhod, could raise some concerns. Big data analytics could also predict that people that do not participate in social media are 30% more likely to be identity thieves, leading to a fraud detection tool to flag them as “risky” before granting them a loan or another financial benefit or service. When using data for eligibility decisions, organizations should be, at the very least, transparent. When organizations are using trends or some type of consumer scores or statistics in order to apply a decision towards an individual, they should keep in mind that they would need to comply with PIPEDA’s data quality principle as well as consider human rights laws which may apply. There was an interesting finding in PIPEDA Report of Findings #2012-005 (Ontario insurance company used credit information to assess risk; calculate premiums) which summarizes the concerns with transparency and data accuracy when using scores for insurance underwriting purposes.
Another issue to consider is whether the use of the aggregated data (or trend) may bring ethical concerns. Some could raise such concerns with targeted advertisement for financial products for low income consumers who may otherwise be eligible for better offers (and who may never receive them). The May 2016 White House Report “Big Data: A Report on Algorithmic Systems, Opportunity, and Civil Rights” raises that Big data is here to stay, and that the question now is how it will be used: to advance civil rights and opportunity or to undermine them.
This content has been updated on May 2, 2024 at 15 h 08 min.